bdm::ARX Class Reference
Linear Autoregressive model with Gaussian noise. More...
#include <arx.h>
Inheritance diagram for bdm::ARX:
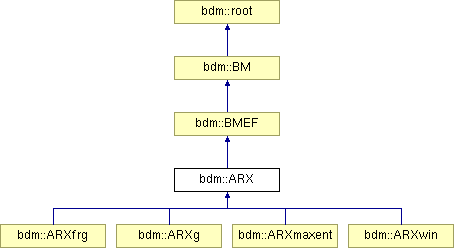
Public Member Functions | |
void | set_statistics (const BMEF *BM0) |
Set sufficient statistics. | |
void | from_setting (const Setting &set) |
void | validate () |
void | set_prior (const epdf *prior) |
function sets prior and alternative density | |
void | set_prior_default (egiw &prior) |
build default prior and alternative when all values are set | |
void | to_setting (Setting &set) const |
RV & | _rgr () |
access function | |
bool | _have_constant () |
int | _rgrlen () |
Constructors | |
ARX (const double frg0=1.0) | |
ARX (const ARX &A0) | |
ARX * | _copy () const |
void | set_frg (double frg0) |
void | set_constant (bool const0) |
void | set_statistics (int dimy0, const ldmat V0, double nu0=-1.0) |
Mathematical operations | |
void | bayes_weighted (const vec &yt, const vec &cond=empty_vec, const double w=1.0) |
Weighted Bayes ![]() | |
void | bayes (const vec &yt, const vec &cond=empty_vec) |
double | logpred (const vec &yt, const vec &cond) const |
vec | samplepred (const vec &cond) |
void | flatten (const BMEF *B, double weight) |
enorm< ldmat > * | epredictor (const vec &rgr) const |
Conditioned version of the predictor. | |
estudent< ldmat > * | epredictor_student (const vec &rgr) const |
template<class sq_T> | |
shared_ptr< mlnorm< sq_T > > | ml_predictor () const |
conditional version of the predictor | |
template<class sq_T> | |
void | ml_predictor_update (mlnorm< sq_T > &pred) const |
fast version of predicto | |
mlstudent * | predictor () const |
ivec | structure_est (const egiw &Eg0) |
Brute force structure estimation. | |
ivec | structure_est_LT (const egiw &Eg0) |
Smarter structure estimation by Ludvik Tesar. | |
void | reduce_structure (ivec &inds_in_V) |
reduce structure to the given ivec of matrix V | |
Access attributes | |
return correctly typed posterior (covariant return) | |
const egiw & | posterior () const |
Protected Attributes | |
bool | have_constant |
switch if constant is modelled or not | |
vec | dyad |
vector of dyadic update | |
RV | rgr |
RV of regressor. | |
int | rgrlen |
length of the regressor (without optional constant) | |
egiw | est |
posterior density | |
egiw | alter_est |
Alternative estimate of parameters, used in stabilized forgetting, see [Kulhavy]. |
Detailed Description
Linear Autoregressive model with Gaussian noise.Regression of the following kind:
where unknown parameters rv
are , regression vector
is a known function of past outputs and exogeneous variables
. Distrubances
are supposed to be normally distributed:
See Theory of ARX model estimation for mathematical treatment.
The easiest way how to use the class is:
#include "estim/arx.h" #include <vector> #include <iostream> #include <fstream> using namespace bdm; // estimation of AR(0) model int main(){ //data vector<vector<vector<string>>> string_lists; string_lists.push_back(vector<vector<string>>()); string_lists.push_back(vector<vector<string>>()); string_lists.push_back(vector<vector<string>>()); char* file_strings[3] = {"c:\\ar_normal.txt", "c:\\ar_student.txt", "c:\\ar_cauchy.txt"}; for(int i = 0;i<3;i++) { ifstream myfile(file_strings[i]); if (myfile.is_open()) { while ( myfile.good() ) { string line; getline(myfile,line); vector<string> parsed_line; while(line.find(',') != string::npos) { int loc = line.find(','); parsed_line.push_back(line.substr(0,loc)); line.erase(0,loc+1); } string_lists[i].push_back(parsed_line); } myfile.close(); } } //prior mat V0 = 0.0001 * eye ( 3 ); //V0 ( 0, 0 ) = 0.1; // for(int j = 0;j<string_lists.size();j++) { for(int i = 0;i<string_lists[j].size();i++) { ARX Ar; Ar.set_statistics ( 1, V0 ); //nu is default (set to have finite moments) Ar.set_constant ( false ); Ar.validate(); // forgetting is default: 1.0 vector<vec> conditions; for(int k = 1;k<string_lists[j][i].size();k++) { vec condition; condition.ins(0,string_lists[j][i][k]); conditions.push_back(condition); if(conditions.size()>1) { conditions[k-2].ins(0,string_lists[j][i][k]); } if(conditions.size()>2) { conditions[k-3].ins(0,string_lists[j][i][k]); //cout << conditions[k-3] << endl;// << conditions[k-3].left(1) << conditions[k-3].right(2); Ar.bayes(conditions[k-3].left(1),conditions[k-3].right(2)); } } ofstream myfile; myfile.open("c:\\classic_ar1.txt",ios::app); myfile << Ar.posterior().mean()[0] << ";"; myfile.close(); myfile.open("c:\\classic_ar2.txt",ios::app); myfile << Ar.posterior().mean()[1] << ";"; myfile.close(); } ofstream myfile; myfile.open("c:\\classic_ar1.txt",ios::app); myfile << endl; myfile.close(); myfile.open("c:\\classic_ar2.txt",ios::app); myfile << endl; myfile.close(); } }
odo sort out constant terms - bayes should accept vec without additional 1s
Member Function Documentation
void bdm::ARX::from_setting | ( | const Setting & | set | ) | [virtual] |
Create object from the following structure
class = 'ARX'; rgr = RV({'names',...},[sizes,...],[times,...]); % description of regressor variables --- optional fields --- prior = configuration of bdm::egiw; % any offspring of eqiw for prior density, bdm::egiw::from_setting alternative = configuration of bdm::egiw; % any offspring of eqiw for alternative density in stabilized estimation of prior density constant = []; % 0/1 switch if the constant term is modelled or not --- inherited fields --- bdm::BMEF::from_setting
prior = posterior; % when prior is not given the posterior is used (TODO it is unclear)
alternative = prior; % when alternative is not given the prior is used
constant = 1; % constant term is modelled on default
Reimplemented from bdm::BMEF.
Reimplemented in bdm::ARXg.
ivec bdm::ARX::structure_est | ( | const egiw & | Eg0 | ) |
Brute force structure estimation.
- Returns:
- indices of accepted regressors.
ivec bdm::ARX::structure_est_LT | ( | const egiw & | Eg0 | ) |
Smarter structure estimation by Ludvik Tesar.
- Returns:
- indices of accepted regressors.
The documentation for this class was generated from the following files:
- arx.h
- arx.cpp
Generated on 2 Dec 2013 for mixpp by
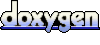